Understanding the potential effects of hurricanes before they strike is crucial for helping residents prepare and take necessary actions, such as evacuation. Researchers at MIT have developed an innovative approach that combines generative artificial intelligence (AI) with a physics-based flood model to simulate future satellite images of areas affected by flooding. This breakthrough aims to enhance preparedness and improve decision-making in risk-sensitive scenarios.
The Earth Intelligence Engine: An Overview
The new technique, named the “Earth Intelligence Engine,” produces realistic satellite images of potential flood-prone regions by combining data from generative AI and physical flood simulations. While traditional flood forecasting tools rely on colour-coded maps to show possible inundation, this method offers a visual layer that is more intuitive and relatable, enabling individuals to better grasp the risks within their communities.
Also Read – Keeping Up with the Times: Applying GIS Tools for Flood Disaster Mitigation
The process starts with a generative adversarial network (GAN), a machine learning model designed to create realistic images by utilizing two neural networks working in tandem. These networks refine their outputs by analyzing real satellite images captured before and after hurricane events. However, the addition of a physics-based model is what sets this technique apart, as it incorporates variables like hurricane trajectory, wind strength, and storm surge to ensure that the images accurately reflect real-world conditions.
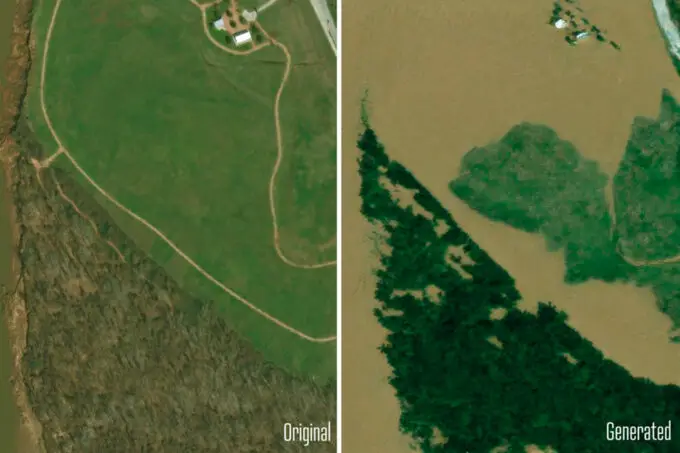
How the Technology Works
The core of this groundbreaking approach lies in the integration of Generative Adversarial Networks (GANs) and physics-driven simulations.
Generative Adversarial Networks (GANs): A Creative Engine
GANs are a type of machine learning framework composed of two neural networks, working in tandem to create highly realistic synthetic images:
- Generator Network: Trains on pairs of satellite images captured before and after flooding events to learn patterns of flooding. It uses this knowledge to generate new satellite images of predicted flooding for specific regions.
- Discriminator Network: Evaluates the generator’s outputs, comparing them to real satellite images and identifying discrepancies. This feedback loop improves the quality of generated images over time.
However, GANs alone can sometimes produce errors, such as depicting flooding in areas that are physically impossible, a phenomenon referred to as “hallucinations.
Also, Read – GIS Resources Magazine – Geospatial Technology to Mitigate the Impact of Flooding
Physics-Based Flood Model: Adding Real-World Accuracy
To address these inaccuracies, the GAN is paired with a physics-based flood model. This model incorporates critical real-world variables, such as:
- Hurricane Track and Intensity: Determines the storm’s trajectory and power.
- Wind Patterns and Storm Surge: Simulates how wind pushes water onto land.
- Hydraulic Infrastructure: Maps the local drainage systems and barriers to predict how water flows and accumulates.
The physics model generates flood predictions that align with the physical characteristics of the landscape. When integrated with the GAN, this ensures the final satellite images reflect real-world conditions, eliminating erroneous outputs.
Testing the Concept
To validate the method, researchers used data from Houston, simulating scenarios based on Hurricane Harvey, which caused extensive flooding in 2017. The team compared the AI-generated flood visuals with real satellite images of the same areas after the storm. They found that the physics-enhanced approach significantly improved the accuracy of the predictions, avoiding the “hallucinations” often seen in standard AI models, where flooding is depicted in physically impossible locations.
While this proof-of-concept study highlights the effectiveness of combining machine learning with physics-based models, broader implementation will require further training on diverse regional data to account for varying topographies and environmental conditions.
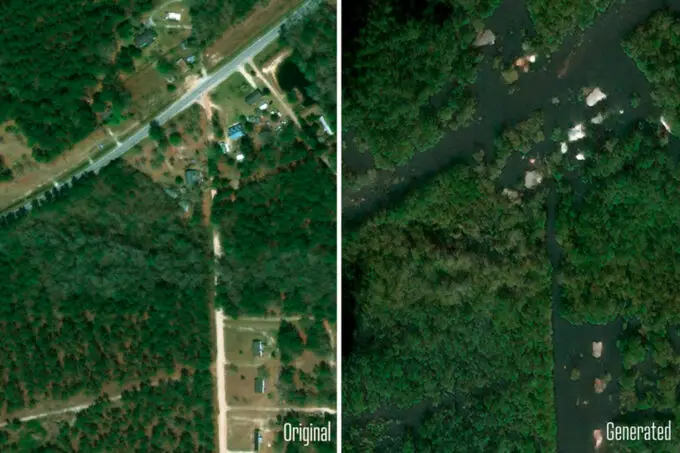
Practical Applications and Future Potential
The goal of this project is to make flood simulations more accessible and actionable. By visualizing potential flood impacts in satellite imagery, local communities and policymakers can better understand and address risks. This approach may also help improve evacuation compliance by making the dangers of an approaching storm more tangible.
The researchers have made the Earth Intelligence Engine available as an online tool for experimentation and collaboration, signalling its potential as a resource for future climate impact studies. Beyond flooding, the technique could be adapted to visualize other climate-related threats, such as wildfires or droughts, making it a versatile tool for disaster preparedness.
Conclusion
This groundbreaking method from MIT demonstrates how advanced technology can address real-world challenges, merging artificial intelligence with physical science to produce accurate, trustworthy data. By enhancing how communities visualize and prepare for natural disasters, this innovation could play a critical role in safeguarding lives and property. With continued research and broader training, the Earth Intelligence Engine could become an indispensable tool for mitigating the impacts of climate change.
Source: MIT