About Author
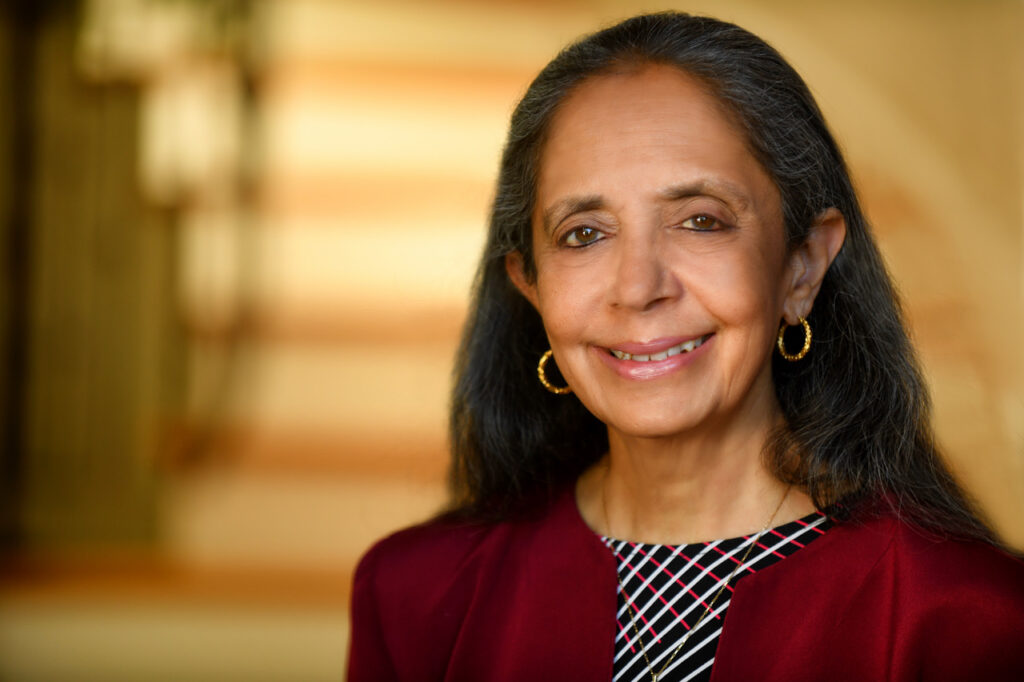
Radha Basu
Founder and CEO, iMerit
Radha Basu is the Founder and CEO of iMerit, a global AI
data solutions company delivering high-quality data that
powers machine learning and artificial intelligence
applications for Fortune 500 companies.
Thousands of years ago, farming started on a small scale to provide food for smaller groups. Eventually, as civilizations evolved, farmers began cultivating the land to provide food for larger communities. They faced several challenges to achieve higher yields and productivity. However, throughout, there were visionaries and technological revolutions that made fields more bountiful. From new irrigation processes to better crop management, farming became a steadily more scientific enterprise that benefited people across the globe.
Challenges in Traditional Farming
Soil quality, rainfall, temperature, humidity, quality of seeds and many other elements play an important role in farming. Changing weather patterns and environmental impacts are drastically affecting these elements, making it difficult for farmers to predict and make decisions related to preparing the soil for sowing, predicting rainfall and other weather changes, identifying the right land and crops to grow, preparing for birds and animal migration to protect crops, and more.
Traditionally, farmers invested a lot of time, money and effort to make the right decisions leading to a good yield. It also delayed farming processes and affected eventual outputs negatively.
Role of Artificial Intelligence (AI) and Technology
Farmers and agricultural solutions providers are turning to modern technologies to achieve a healthier yield, monitor soil health and track crop growth. This is not only helping farmers to reduce their workload but is also enhancing the quality of the produce. Today, the agriculture sector is applying AI to a wide range of farming tasks in the food supply chain.
- Real-time updates: Using image recognition technology based on deep learning, farmers can automate detection of plant diseases and pests. These methods classify, detect and segment images to build intelligent models to keep an eye on crop health, which was traditionally done by people. This can also be used to get real-time updates on crop well-being or to identify human or animal breaches affecting the crops.
- Pest and weed management: AI systems are capable of using satellite images and historical data to detect and predict possible attacks by insects and the type of insects like locusts, grasshoppers, etc and thus provide precision agriculture. On detecting a possible pest infestation, these systems can alert the farmers through their smartphones so that the farmers can take the right decision at the right time. Similarly, AI can help in differentiating weeds from crops or identify diseased crops so that they can be cleared.
- Intelligent spraying: Smart sensors, powered by AI, on detecting weed or pest-affected areas, can automate the spraying of herbicides and pesticides in the right quantity in the right places. These systems can be built in unmanned aerial vehicles installed with sprayers that can operate with high precision in terms of the area and amount to be sprayed. These can cover larger areas, which, done traditionally, can take a lot of time and manpower.
- Monitoring weather and soil conditions: Change in climatic conditions and fluctuations in the quality of air can drastically impact crop health. It can also lead farmers to make wrong decisions, affecting yields. With the help of AI systems and precision agriculture techniques, farmers can analyze and predict weather conditions to plan the type of crop and time for the seeds to be sown. Likewise, these intelligent systems can analyze soil health and help farmers to determine the right fertilizer and amount of nutrients required to make the soil beneficial for the crop.
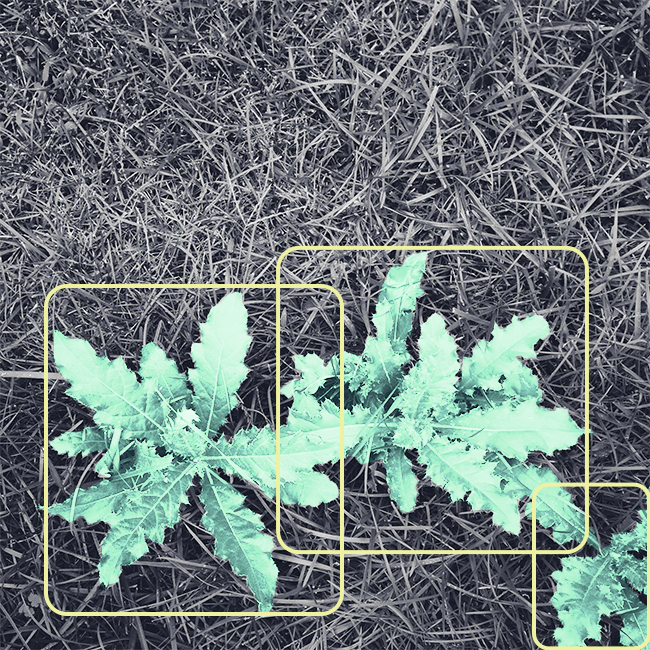
Artificial intelligence can help farmers to automate processes and empower them to cultivate for higher crop yield, quality and faster output while using fewer resources.
GIS in Precision Agriculture
While farmers seek technology to analyze and measure efficiency of their fields, they can increasingly benefit from a bird’s eye view of all the farmlands to gather deeper insights. This geospatial data has the potential to advance farming and optimize resources. GIS-driven models enable farmers to evolve and develop more efficient techniques.
The use of remote sensors is not limited to large farmlands. Even small farms can adopt methods such as zone-based field management, which splits fields into smaller sections based on the type of crops, type of soil composition and many other factors.
The precision agriculture concept does not consider a farm as a homogenous entity; it factors in critical variations in soil composition, nutrients in that soil and even moisture levels and quality. All of that requires consistent measurement through the toolsets available to geospatial data analysts, and the consequent use of all available means of acquiring that data (remote sensors, satellite imagery, drone and other aerial imagery, GPS reckoning, etc.).
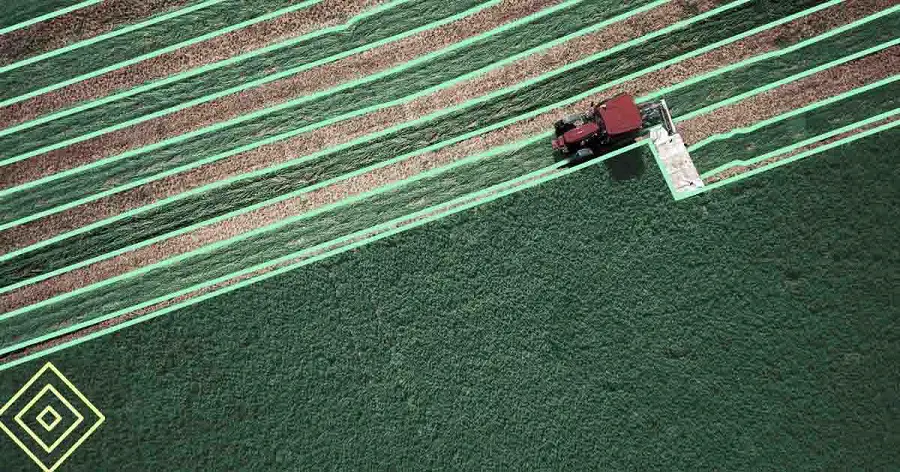
With GIS availability, factors such as pH rates, the presence of pest infestation, types of nutrients found in the soil (and consequent need for intervention with specific fertilizers), the crops – and their characteristics, including health and density – and even overlaying weather forecasts are consistently monitored and analyzed to make better decisions.
Importance of High-quality Data
GIS and other geospatial techniques for precision agriculture use data around historical land use and characteristics, often supplied by government agencies in the form of survey maps and records, as well as more current data such as infrared and photographic imagery picked from both public and private sources.
Precision agriculture thus relies on image-based data from remote sensing such as determining the greenery of the farmland using a technique to determine the productivity or yield of different zones. This technique is based on the relationship that arises from the comparison of the reflection of red light and near-infrared light.
Data acquisition also needs to account for seasonal and situational changes that add substantially to the requirements for data analysis. Over a period of time, the collected data can provide a precise picture of crop productivity at a granular level as well as growth and yield patterns over the years, all adjusted for seasonal fluctuations. This process is deeply reliant on both the quantity and quality of the data acquired from various sources. It enables analysts to label and organize data to deliver accurate results accurately. Whether the end consumer is a farmer or AI algorithm developer, both groups need accurate annotation of the data as well as the consistency crucial to pattern recognition.
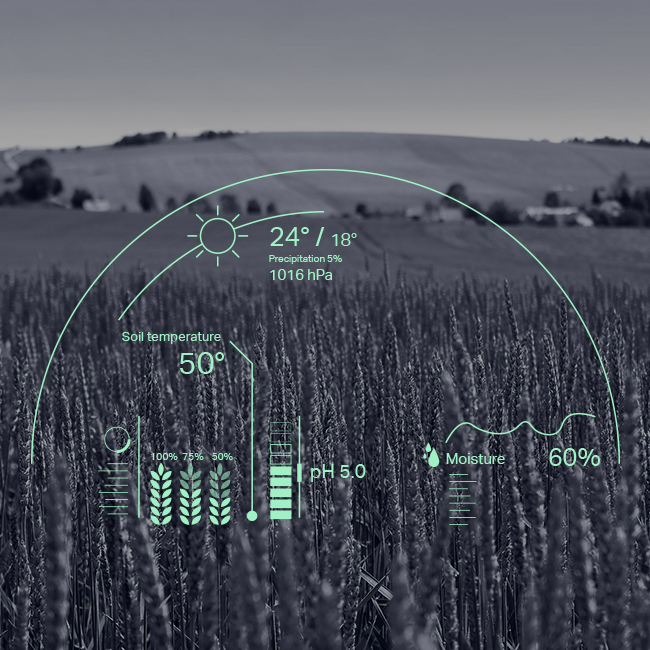
Challenges in Annotating Crops
Annotating crops can be surprisingly challenging as technology-driven systems lack the human intelligence to sort the various varieties that might have similar features. For example, many variations of wheat, to a relatively untrained eye, can look like weeds – or vice versa. Subsequently, training an algorithm to approximate the knowledge and experience of a farmer requires a considerable amount of data, which can be overwhelming.
More data and diverse data points are always helpful to accelerate algorithms to learn more and aid in decision-making for farmers or scientists. It is clear to many in the sector that a multi-year development project to provide training data to an algorithm for field machinery could require semantic segmentation on hundreds of thousands of images before a farming operation would be able to move from development to live production technology. This is not a simple task.
How Experts Can Help
Understanding GIS and geospatial techniques and implementing them efficiently to enhance agriculture need human-in-the-loop practice to feed, train and monitor algorithms. A data annotation and analysis company such as iMerit will assemble a team of dedicated data analysts to take one or more of a series of annotation-related tasks such as annotating raw images, conducting quality checks (QC) on pre-annotated images, or even some secondary QCs. Combining annotation and verification as a client service can cut costs significantly, up to 50%, compared to other options.
Sentera, a global leader delivering time-sensitive agricultural insights, needed annotation support to scale its business for critical opportunities. Sentera’s deep-learning platform FieldAgent helps farmers use drones to gather data and generate real-time insights around weather, soil, and field operations to improve harvests, inform growth strategy, and control pests. One of Sentera’s customers was consulting with farmers who were planting 30,000 plants of corn per acre and wanted to use drones to monitor crop growth progress.
Sentera chose iMerit for their tool-agnostic approach, integration with AWS SageMaker Ground Truth and expert human-in-the-loop annotation. iMerit began the project by successfully annotating test batches around Sentera’s edge cases. These results helped Sentera realize how they could leverage iMerit to improve the deep-learning outputs of FieldAgent. iMerit’s feedback helped Sentera create unambiguous and actionable instructions for annotators across the image data to ensure every tassel is labeled. After iMerit annotated 1.2 million tassels with 95% accuracy, training datasets were created and fed into FieldAgent’s algorithm to improve tassel detection.
The agriculture sector has become one of the biggest consumers of GIS and geospatial data across the globe. Data annotation specialists have thus stepped up to play a pivotal role in enriching GIS images and unearthing ground realities to help the sector reduce costs and enhance yields. Additionally, by employing technology, farmers and agriculture businesses can achieve more with less resources. GIS and geospatial intelligence is consequently capable of solving global food supply chain problems by supporting agriculture practices, especially in regions facing famine, droughts or other challenges.
Also Read –
How AI and Aerial Imagery Can Improve Crop Damage Assessments in Agricultural Insurance